- Data Center
- Blaize
The Quest for More Sustainable and Efficient Enterprise and Edge Data Center AI Computing
The enterprise and edge data center landscape is undergoing a substantial transformation driven by the rapid adoption of AI and the increased amount of data generated and consumed outside the traditional cloud and on-prem boundaries.
IDC research shows that today, nearly 80 zettabytes (ZB) of data are generated at the enterprise edge. This volume continues to grow and is projected to rapidly exceed 150 ZB in the next few years1.
Business is becoming increasingly decentralized. As data multiplies, enterprises realize the benefits of bringing AI capabilities closer to the data source, valuing high performance, low latency, and data locality. Garner reports that 50% of critical enterprise applications will reside outside of centralized public cloud locations. Also fueling this are common trends across industries adopting AI, such as users wanting to maintain control of their data, desire for independence from the cloud, isolation of operation due to security or logistical reasons, and the growing adoption of data-centric AI applications.
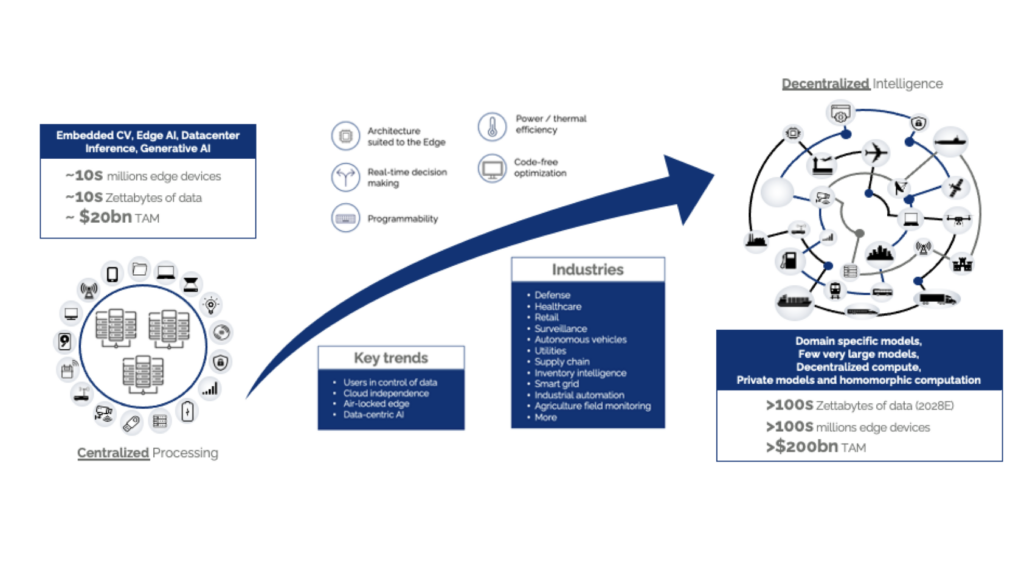
Image: A world moving toward AI everywhere
Let’s look at some key trends and issues driving the industry’s appetite for a different approach.
Sustainability and energy-efficient computing
Making data centers of all sizes run more efficiently is imperative to meet sustainability goals.
Data centers are estimated to consume up to 3% of global electricity today2, growing to 4% by 2030. For scale, the average facility (approx. 20-50MW per year) could power up nearly 40,000 homes3 and requires 11-19 million liters of water per day for cooling (a similar capacity used by a city with 30-50,000 people)4. In fact, cooling accounts for 40% of the total energy consumption5. Power-intense workloads such as AI and adopting power-hungry GPUs (Graphics Processing Unit) servers are key contributors. The market needs alternative architectures to deliver more compute density and do the same job more efficiently.
We are not talking only about cloud hyperscalers and large enterprises on-prem facilities. Small data centers (server closets, small IT rooms, and localized data centers under 500 square meters and budgeting less than 100-150 kW) make up more than half the total electricity consumption worldwide6. Reducing the energy consumption of these local at-the-edge deployments would translate into a significant global sustainability benefit.
Furthermore, micro data centers or MDCs (small-scale modular units that include all the compute, storage, networking, power, cooling, and other infrastructure – typically a stand-alone single deployment unit, a single or small number of racks) are gaining traction where it is impractical to deploy traditional data centers, such as in hospitals, warehouses, smart factories, remote locations, and the like. MDCs can offer significant sustainability benefits by placing resources closer to the data, reducing the energy needed to transfer information across a network, and reducing the cooling and power costs associated with larger centralized solutions.
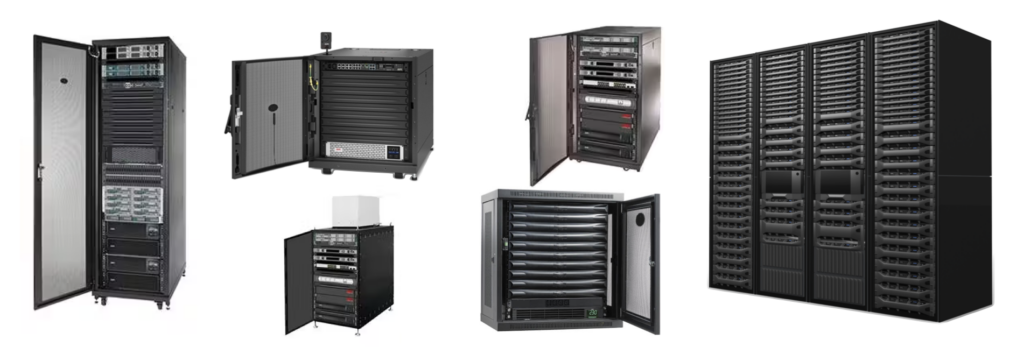
Image: Examples of various micro data center (MDC) configurations. MDCs are small, self-contained data centers that house a complete data center infrastructure in a single space and help implement edge computing in the real world.
Incumbent inference solutions are not cost-effective
Inference exceeds the costs of training and accounts for up to 90% of the machine learning costs for deployed AI systems. Over 90% of on-prem and edge deployments are anticipated to be for inference in the future, while enterprises are expected to carry out most (>80%) of all training on the cloud7. The data center inference chip market is growing much faster and is forecasted to reach $35bn by 2025, compared to $10.1bn for training. Inference data center allocations are expected to grow at much faster rates than training8.
The GPU continues to be the workhorse for AI training, albeit alternative solutions are gaining traction from merchant vendors or in-house designs (hyperscalers)9. While GPUs excel at training, they are overengineered for inference loads and could be more cost-effective. They are expensive10 and primarily architected for training, which adds complexity (higher precision accumulation, more emphasis on data movement and synchronization, larger on-chip caches, etc). The off-the-shelf costs associated with leading-edge GPU servers also can quickly add up.
As AI transforms the economy, enterprises will increasingly deal with specialized and fine-tuned smaller models, often on private data, and with multimodality (models that can process and understand various data formats like images, audio, and video). New architectures must be flexible, scalable, and designed for programmability and futureproofing to keep pace with the fast-changing characteristics and requirements of AI workloads and applications.
Many dedicated AI accelerators and architectures in the industry today carry a legacy burden. CPUs (Central Processing Units) were designed for general purpose applications such as web browsers and productivity, while GPUs were for gaming and graphics. These processors are being extended and improved also to do AI, but they were not designed for it. Many dedicated AI hardware vendors focus on achieving headline performance points by specializing on a small subset of applications via fixed function hardware (common in deep edge video analytics).
In summary, inference is king in enterprise AI deployments. CPUs, GPUs, and dedicated hardware accelerators today are not the most efficient or cost-effective approach.
More control over hardware and software
Enterprises and industries adopting AI seek more control over technology, from hardware to software.
GPU vendors and other incumbents provide proprietary software APIs and tightly controlled ecosystems of partners, resulting in unwanted vendor lock-in. Developers and software vendors value the flexibility and power of programming any target accelerator without the stickiness of proprietary APIs. They prefer using portable industry-standard APIs, open software frameworks, and turn-key integration with their ISVs of choice.
Porting applications or adopting and deploying new AI use cases can be complex and time-consuming. Historically, enterprises had to resort to expensive data scientists and teams of programmers to resolve this. There is an appetite in the industry for novel approaches that empower subject experts who are not coders or data scientists to express their needs to the AI tool more accessible and unlock the value of AI for their applications faster.
All industries adopting AI are searching for reduced costs and more control over technology, leading to a more dynamic and diversified market for AI accelerators. Enterprises want to be able to choose the right fit for their needs.
Enterprises are also becoming sensitive to the financial impact of using AI accelerators over their entire lifespan, not just the upfront costs of purchasing the hardware. This includes all associated expenses that come with owning and operating the accelerators. There is an appetite in the industry for more efficient hardware that requires less cooling, does not include a brand premium, is easy to use, has low complexity and deployment barriers, has flexible business models, and has no vendor lock-in. In short, a more compelling total cost of ownership is now a critical decision factor.
Coming up
The rapid adoption of AI and the explosion in the volume of data generated and consumed outside the traditional cloud and on-prem boundaries are driving the need for efficient decentralized AI compute solutions at the edge across most industries.
Data centers of all sizes, from micro deployment to large enterprises and cloud, consume a lot of energy. Enterprises and industries are hungry for more efficient and sustainable solutions. Most deployed AI consists of inference processing, and incumbent inference accelerators are not cost-effective. Other industry concerns are the total cost of ownership and avoiding vendor lock-in.
In our next blog, we will explore how Blaize solutions address the needs of the enterprise and edge data center market. We will examine the hardware characteristics and how our unique software solutions add value. Future articles will explore practical industry use cases in retail, enterprise, and defense verticals.